Data modernization and moving to the cloud. Common challenges and pitfalls
Read the article below or download the PDF version here.
In the evolution to a data-centric organization, businesses continually strive to modernize their data infrastructure and unlock the full potential of data assets. In this constant striving for productivity gains and cost control, separating the benefits of data modernization from platform renewal as separate projects will result in only partial achievement of the goal to build and maintain a competitive, or comparative advantage through data.
When asked about data modernization strategy, many firms will include and sometimes focus on, cloud migration projects. Scalable storage, processing, and infrastructure are essential to handle growing data volumes and compute requirements but it is also critical to improve the underlying asset, the data. Lifting and shifting to new technology may resolve performance impediments of legacy systems but does not address the fundamental issue of improving the quality and utility of the data. Successful data modernization involves assessing and meeting the future needs of data users for reporting, analysis, innovation, and workflow management.
Together, the technology and data modernization process are the foundation to enabling organizations to build and scale flexibly and cost-effectively. It is the driver of creativity and connectivity, the underlying requirements for organizational success.
While this modernization program will deliver numerous benefits, it is not without its challenges.
In this blog article, Peter Jones, Chief Product Officer at RoZetta Technology, outlines common challenges and pitfalls that organizations encounter during their data modernization journey.
“Never view obstacles in your path as the enemy. Rather, view any obstacles as detour signs to avoid pitfalls.”
-Donald L Hicks
Start with WHY?
It is important to establish and maintain a focus on why the platform and data modernization program is important. Some key strategic drivers are:
- Data volumes are increasing exponentially, and new platforms are needed to manage the volume and complexity.
- The need to scale and cater for advanced analytics and data science to leverage machine learning and artificial intelligence capability.
- Legacy systems have limitations which inhibit both growth and innovation.
- Data sources are becoming more diverse, particularly with the emerging importance of unstructured data.
- Increasing need to manage regulatory, compliance and governance issues centrally.
- Once the strategic drivers are established keep them in front of the project team as a constant reminder as to ‘why’.
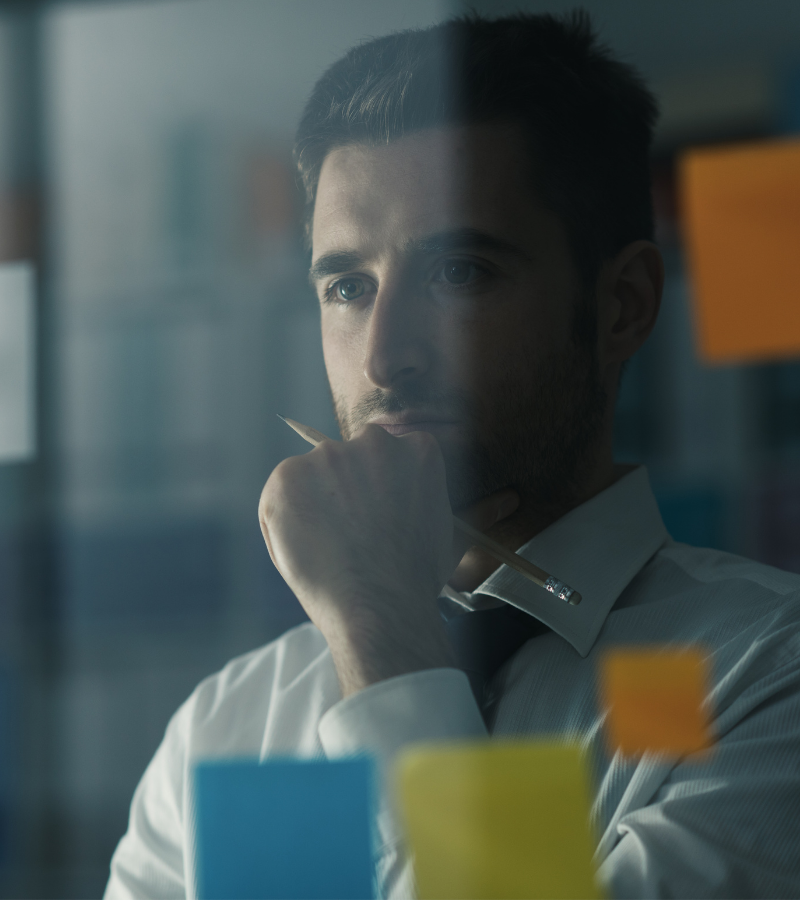
Key process and considerations
The consideration set for data and platform modernization are consistent. The breadth of some component tasks may change based on the current and target state for both data and platforms:
- Assessment & Planning
- Data Profiling & Cleaning
- Data Mapping & Conversion
- Data Migration
- Testing & Validation
- Security & Governance
- Training
- Change Management
- Monitoring & Optimization
Common challenges and pitfalls
In the planning stage of these projects, there are challenges and pitfalls that the project team will want to document and resolve. Ensuring the proposed solution takes these into account will result in a more focused project plan, less scope creep and clarity in expectations of time and deliverables.
#1 Fragmented silos of data
Fragmented data silos within organizations present several challenges around identifying data sources, end-user models, and data consumers across different departments and systems, each with varied data formats and architecture.
Auditing and cataloguing data assets, licenses, and permissions are necessary to gain a comprehensive understanding of the data landscape.
One of the key challenges is addressing a central driver of the project – the standardization, normalization, and unification of data assets into a cohesive data ecosystem.
Selecting and implementing data integration tools that handle various formats and manage large-scale data transformations is far less risky than assigning data engineers to code up the migration from scratch.

Common pitfalls in setting up an efficient data migration project include:
- targeting too much data at each stage; this leads to prioritization issues and can overwhelm a project team.
- failing to analyze or cleanse data before migrating it to a new architecture, which perpetuates existing source data problems in a new technical environment.
- lack of legacy and target data and technology environment expertise. Not having expertise in legacy systems risks retention of multiple platforms for ongoing data asset onboarding, management, analysis, and discovery.
These risks to the project are most common where business units use different data and technology platforms, especially prevalent if an organization has acquired other entities and not integrated them into a core system.
#2 Quality and consistency of data

While facing these challenges some common pitfalls are:
Inconsistent data quality is a fundamental challenge in migration projects, inevitably these arise in organizations with a long history of incremental development across product and operational systems. Managing and enhancing data consistency is crucial for making informed business decisions and driving successful data-driven initiatives. Building trust in the data will result in greater trust in the decisions made using the data.
Integrating complex data from multiple sources and systems will present real challenges around the systematic cleaning, deduplication of existing data assets and then how to seamlessly add new sources while managing access and usage permissions. This challenge is complicated by having to interpret and integrate bespoke data formats requiring transformation, particularly when sourced from unstructured data using varying methodologies.
In essence the real challenge is developing continuous monitoring and data profiling and remediation at the time of ingestion.
- using manual data management without automation and appropriate data quality management analytics and tools. Often a short cut is the longest road to success.
- failing to balance SME, data engineering and data science expertise to introduce best practice solutions using appropriate tools to investigate and remediate data quality issues.
#3 Data compatibility and interoperability

Some of the pitfalls of not resolving data incompatibility are:
Data compatibility and interoperability challenges arise due to diverse data formats, models, taxonomies, and schemes from various sources, standardizing to create a unified data ecosystem is a major challenge.
Compatibility issues for seamless data integration and data exchange with third-party systems is a constant challenge. Developing a solution at the redesign stage of data and platform modernization will leave a lasting efficiency and productivity gain.
- continued data loss, errors in time series and historical data sets, and errors in analytics and reporting processes. It will also result in limiting data interoperability post implementation of the modernization program.
- not ensuring adequate SME and data science expertise to identify, resolve and implement data incompatibility issues, this will simply perpetuate inaccurate data sets.
#4 Managing legacy systems

A pitfall that will arise is resistance from owners of legacy systems and technologies include:
Legacy systems often expand over extended periods by many stakeholders, leading to differing technologies, architectures and data frameworks, resulting in a complex technical stack.
It is very challenging to arrive at a clear strategic path for migrating legacy systems into new cloud or hybrid architectures. Having “review and agree” sessions on well-defined business use cases and outcomes will pay great dividends on completion of the project.
Effective coordination among various teams, stakeholders, and business units is crucial, requiring top-down sponsorship, clear scope, and strong change and program management.
- any lack of commitment will complicate the unification process.
Focusing solely on technical architectural changes without considering business outcomes leads to increased project risk and misalignment of technical benefits and business imperatives. Keep asking why?
#5 Addressing growing data needs

A common pitfall related to growing data needs include:
Scalable storage, processing, and infrastructure are essential to handle growing data volumes and analytics. Real-time processing and analysis demand robust computational resources, which traditional data systems may lack.
This is one of the more challenging aspects of data modernization. The requirement is to implement the right technical platform and data architecture to enable data accessibility, availability, and responsiveness of very large data sets and to scale up and down quickly. It is also a challenge to consider what sources, volumes and types of data are likely to emerge.
- a lack of certainty over storage, computing and scalability required by the business over a protracted period.
Developing patches during the post-implementation review to write enhanced data back into systems adds complexity and risk to the modernization program.
#6 Maintaining data integrity

A common pitfall related to data integrity:
Continuous attention to data management is necessary to extract value from the modernization efforts and is essential to ensure data integrity, accuracy, and security throughout the modernized data ecosystem's lifecycle.
Some of the key challenges relate to a commitment to ongoing data management and maintenance to monitor, update, and optimize data assets to meet evolving business needs.
There is also the need to manage data licensing, subscription agreements, reference data, and compliance standards to maintain data integrity.
- overlooking the risk of inadequate data governance and management post-implementation can hinder the realization of business value and outcomes.
#7 Data security and privacy

Data security and privacy common pitfall includes:
Protecting sensitive data and complying with organizational standards and statutory regulations is a critical responsibility of the data management process. Whilst challenging, part of the program must implement robust data security measures, collaborate with compliance teams, and adopt data governance practices to safeguard data privacy while allowing efficient data sharing and utilization.
Handling regional and local data privacy regulations adds complexity to these efforts.
.
- centralizing and transforming data without considering security and privacy measures can lead to increased risk of non-compliance.
Creating synthetic and masked data sets that maintain data characteristics while protecting sensitive information requires collaboration with regulatory teams.
#8 Costs and budgeting

Pitfalls related to costs and budgeting includes:
Aligning data-related expenses with the organization’s budget and strategy is essential. Managing the cost of new data technologies, licenses, and ongoing support can be complex.
It is very challenging budgeting across both capital and operational expenditure plans. Proper planning and a well-structured change management approach will optimize resources and project costs.
The focus should be on accurately estimating, and then optimizing, the total cost of ownership (TCO) is essential.
- insufficient planning leads to higher costs, duplication, and wasted time and effort.
Budget constraints may limit the scope of modernization efforts or create prioritization challenges. A phased approach to data modernization can help manage costs while maintaining momentum.
Summary
Data and platform modernization involves cultural shifts, process changes, and effective stakeholder management. Particularly, effective stakeholder management is crucial to address concerns and maintain buy-in over the term of the program of work.
A successful data modernization strategy is more than a cloud migration project. Effective planning, collaboration, and adherence to data governance and security practices are crucial for achieving value creating, data-driven outcomes.
About DataHex
RoZetta’s DataHex will deliver a turn-key infrastructure bringing best practices to data management, analysis, and data science utilizing cloud technology. The solution ensures clients retain control of their data and ownership of insight, innovation, and IP that comes through easy access to data sets, tools, and services.
DataHex SaaS Platform provides -
- Scalable, repeatable, purpose-built cloud platform and managed service specialized for data analysts, data scientists, and managers of data-dependent workflow.
- Proven platform that de-risks and eliminates bottlenecks in managing large-scale time series data.
- Streamlined data access via a search engine, data mapping, API connectivity, and cloud delivery, enhancing the overall consumer experience.
- Powerful analytic discovery enabling more Data Science time to be spent on analysis rather than cleansing and preparing raw data.
Why RoZetta Technology?
RoZetta Technology believes that fusing data science, technology, and data management is the path to amplifying human experience and knowledge.
Our clients have a deep understanding of the challenges they face. We bring proven capability, experience and a mindset to create products and systems that overcome these challenges and create more value while solving them. No matter how complex, the blend of good data, the right technology, well-crafted design and smart individuals can solve most problems.